How MAD Must We Be? A Robust Test for Identifying Meaningful Differences Using the Mean Absolute Deviation
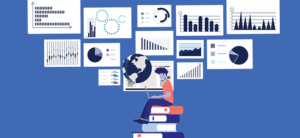
Jon Hasenbank and John Appiah Kubi, Grand Valley State University
This article introduces a method for identifying statistically meaningful differences between two data sets using the mean absolute deviation, known as MAD and a measure of variability taught in middle school curricula under the Common Core State Standards, which parallel the recommendations of the Pre-K-12 Guidelines for Assessment and Instruction in Statistics Education II report.
While students are encouraged to make informal comparative inferences through visual tools and intuitive reasoning, current instructional resources lack precise heuristics to determine when a difference is statistically significant. The authors address this gap by proposing the k-test, a straightforward calculation based on the ratio of the difference in sample means to the pooled MAD, compared against a sample-size-dependent threshold.
Drawing from statistical theory originally developed by Erna Herrey and refined using S. A. Revets’ work on one-norm statistics, the k-test provides a meaningful and accessible alternative to the traditional t-test. Simulation studies reveal that the k-test aligns with the t-test more than 97% of the time, validating its reliability. Unlike the commonly used “2x rule,” which can be overly conservative, the k-test incorporates sample size to yield more accurate conclusions—empowering students and educators to resolve ambiguity in data investigations.
By situating the method within the mathematical knowledge expected at the middle school level, the authors bridge the gap between informal inference and formal statistical reasoning. This approach not only enhances classroom practice by offering clearer decision-making tools but also encourages curiosity about variability, significance, and the role of sample size in statistical analysis.
Download a PDF of the full article.